Abstract
Recent studies have shown that StyleGANs provide promising prior models for downstream tasks on image synthesis and editing. However, since the latent codes of StyleGANs are designed to control global styles, it is hard to achieve a fine-grained control over synthesized images. We present SemanticStyleGAN, where a generator is trained to model local semantic parts separately and synthesizes images in a compositional way. The structure and texture of different local parts are controlled by corresponding latent codes. Experimental results demonstrate that our model provides a strong disentanglement between different spatial areas. When combined with editing methods designed for StyleGANs, it can achieve a more fine-grained control to edit synthesized or real images. The model can also be extended to other domains via transfer learning. Thus, as a generic prior model with built-in disentanglement, it could facilitate the development of GAN-based applications and enable more potential downstream tasks.
Random Walk in Local Latent Spaces
Disentangling Foreground and Background
Our model also disentangles the foreground and background during generation. The user is able to control what to generate for foreground and where to generate it. The location and size of the foreground can be controlled by manipulating Fourier features.
Generation
Shift
Zoom
Generation
Shift
Zoom
Local Style Mixing
Similar to StyleGAN, we can conduct style mixing between generated images. But instead of transferring styles at different granity, we can transfer the styles of different local areas.
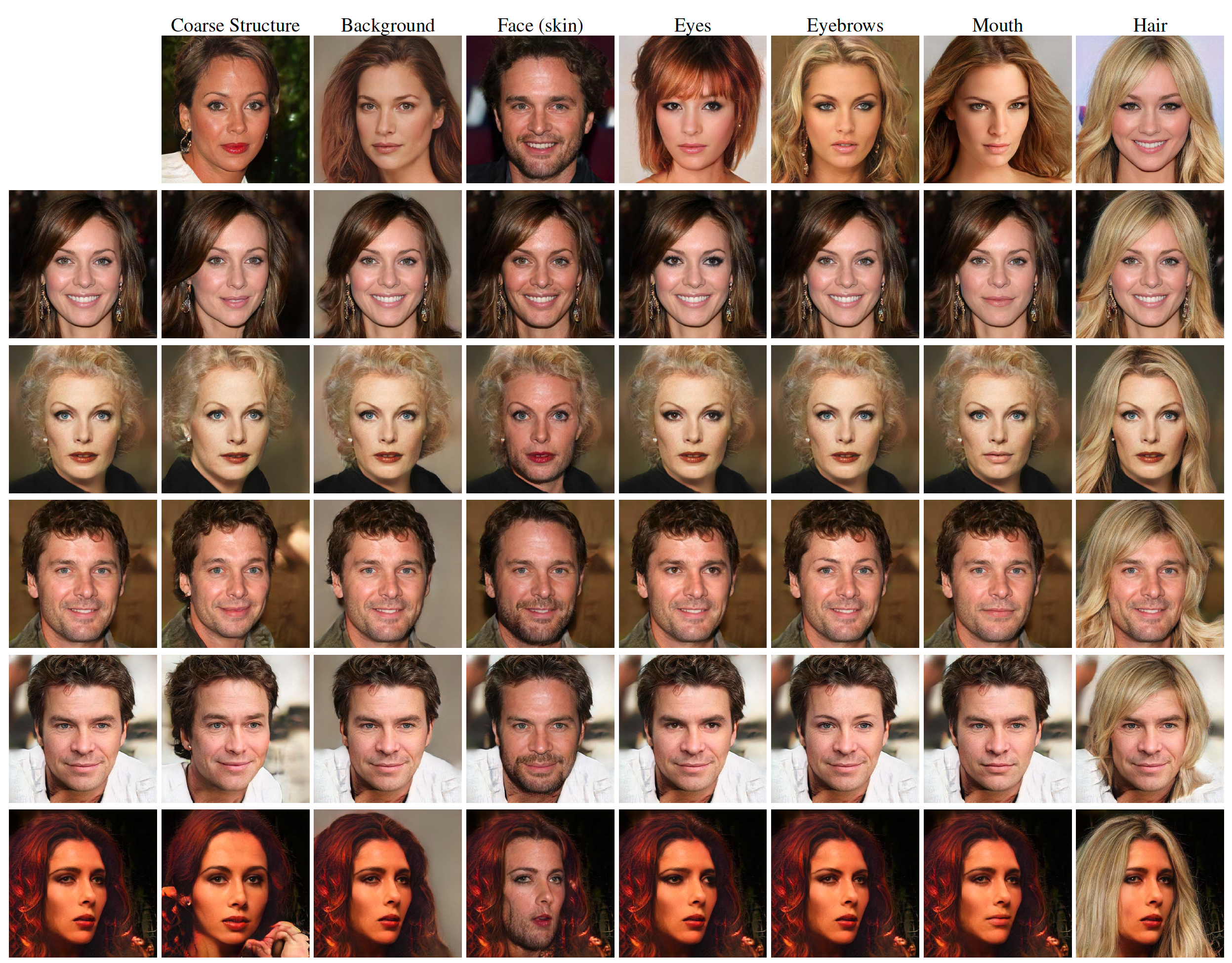
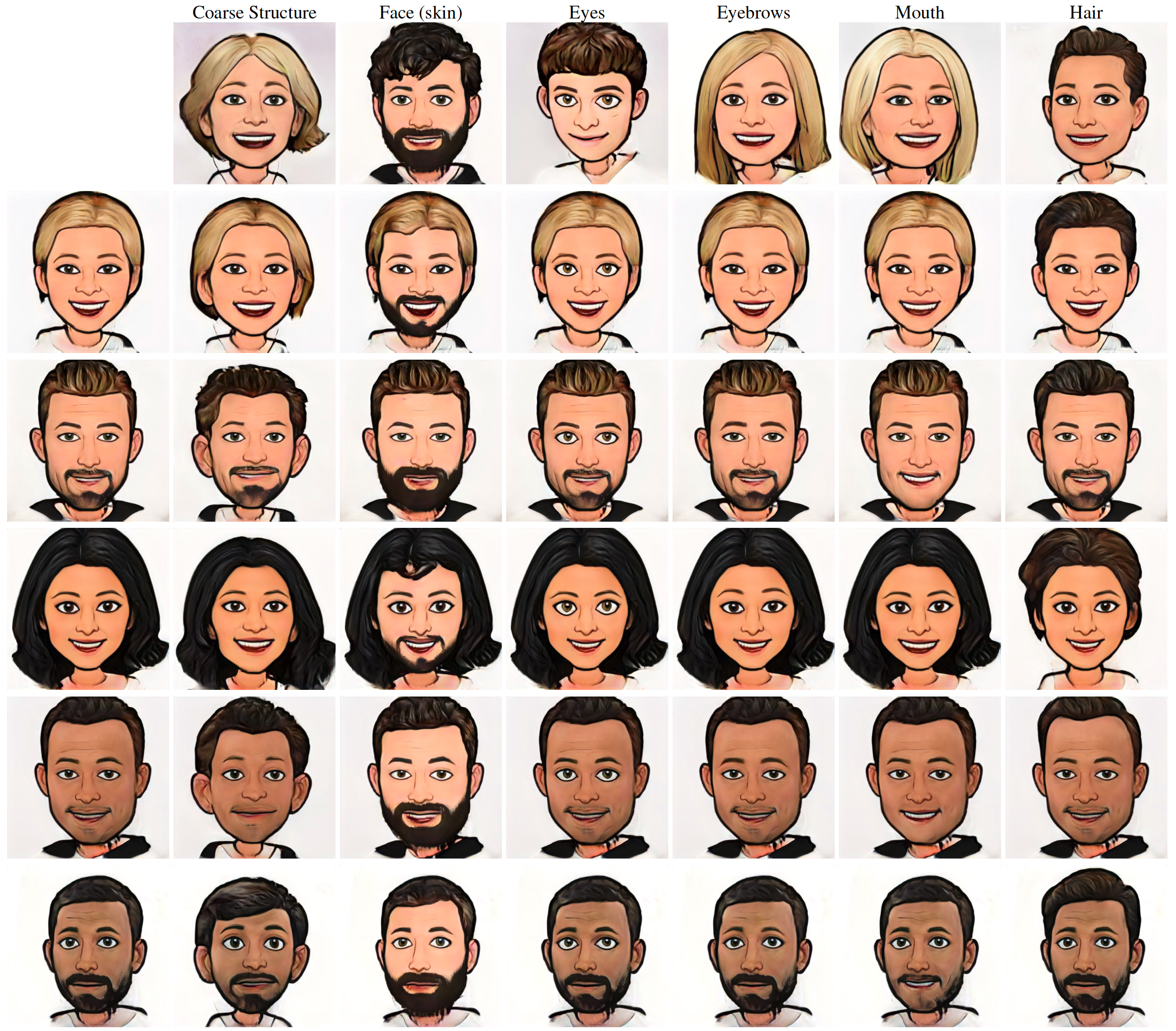
5 Minute Video
BibTex
@inproceedings{shi2021SemanticStyleGAN,
author = {Shi, Yichun and Yang, Xiao and Wan, Yangyue and Shen, Xiaohui},
title = {SemanticStyleGAN: Learning Compositional Generative Priors for Controllable Image Synthesis and Editing},
booktitle = {CVPR},
year = {2022},
}